Multi-Modal Pedestrian Detection with Large Misalignment
Based on Modal-Wise Regression and Multi-Modal IoU
Contributions
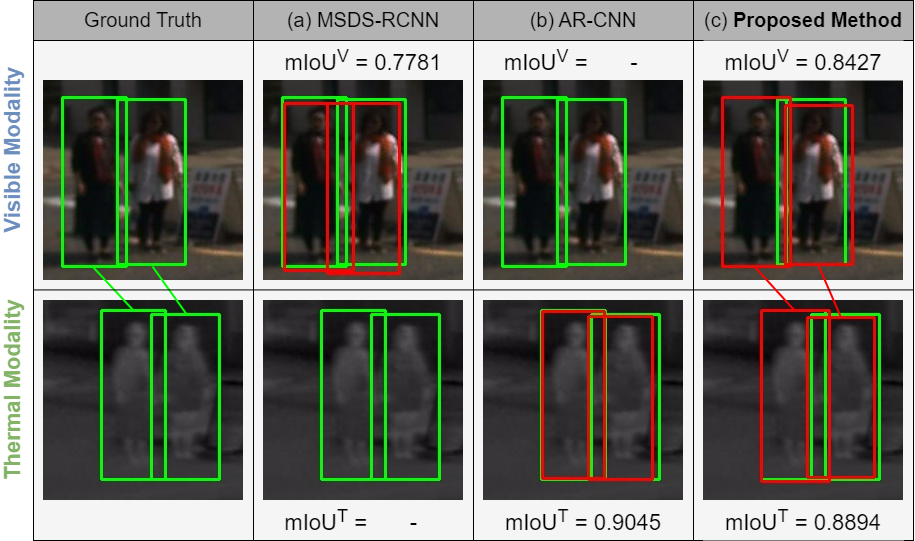
We analyzed the misalignment problem of existing multi-modal detection.
We proposed new evaluation metrics for multi-modal detection, multi-modal IoU (IoUM) and multi-modal MR (MRM).
We proposed multi-modal Faster R-CNN [1] for pedestrian detection based on modal-wise regression and IoUM.
Framework Comparison
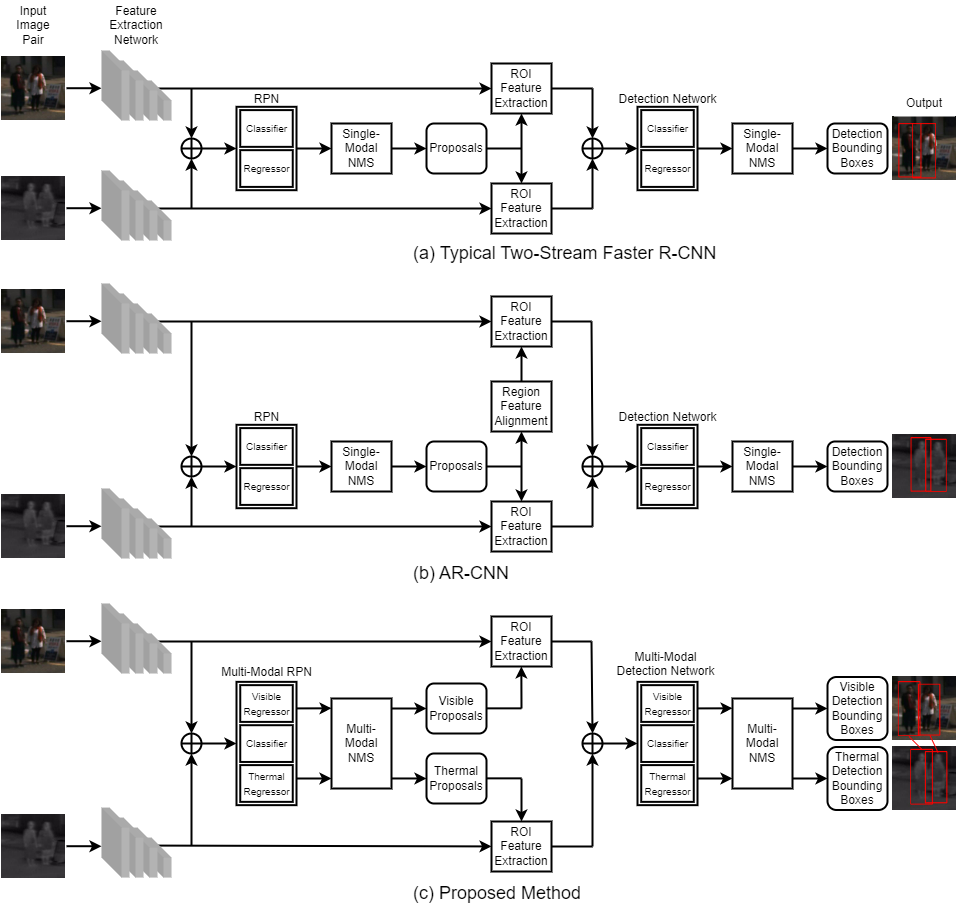
Comparison of multi-modal pedestrian detection frameworks based on faster R-CNN [1].
(a) Typical two-stream faster R-CNN, (b) AR-CNN [4], and (c) proposed method.
Proposed Network Overview
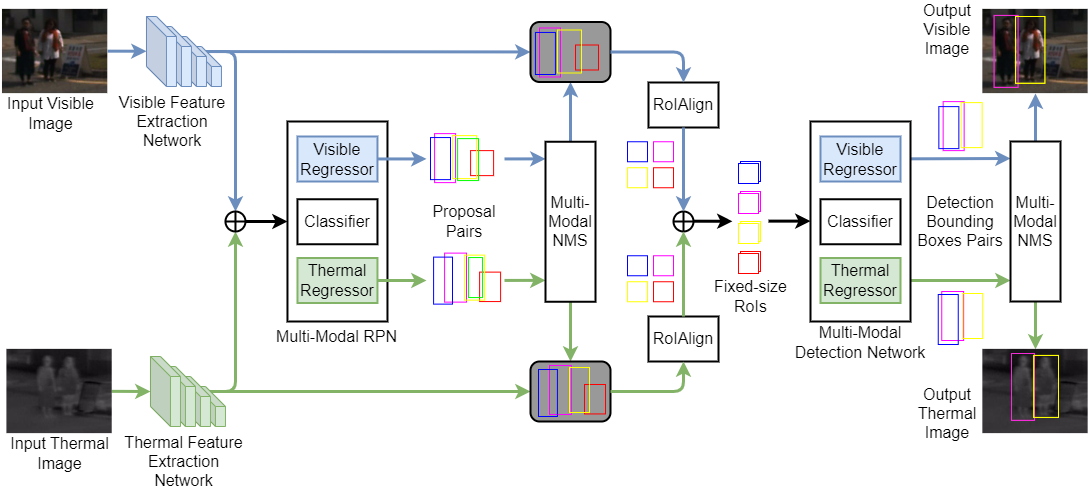
The overall architecture of our network.
We extend Faster R-CNN [1] into a two-stream network to take visible-thermal image pairs as input,
then return pairs of detection bounding boxes as output for both modalities.
Blue and green blocks/paths represent properties of visible and thermal modalities, respectively.
RoIs and bounding boxes with the same color represent their paired relations.
⊕ denotes channel-wise concatenation.
Visualization Examples
MSDS-RCNN |
AR-CNN |
MBNet |
Ours |
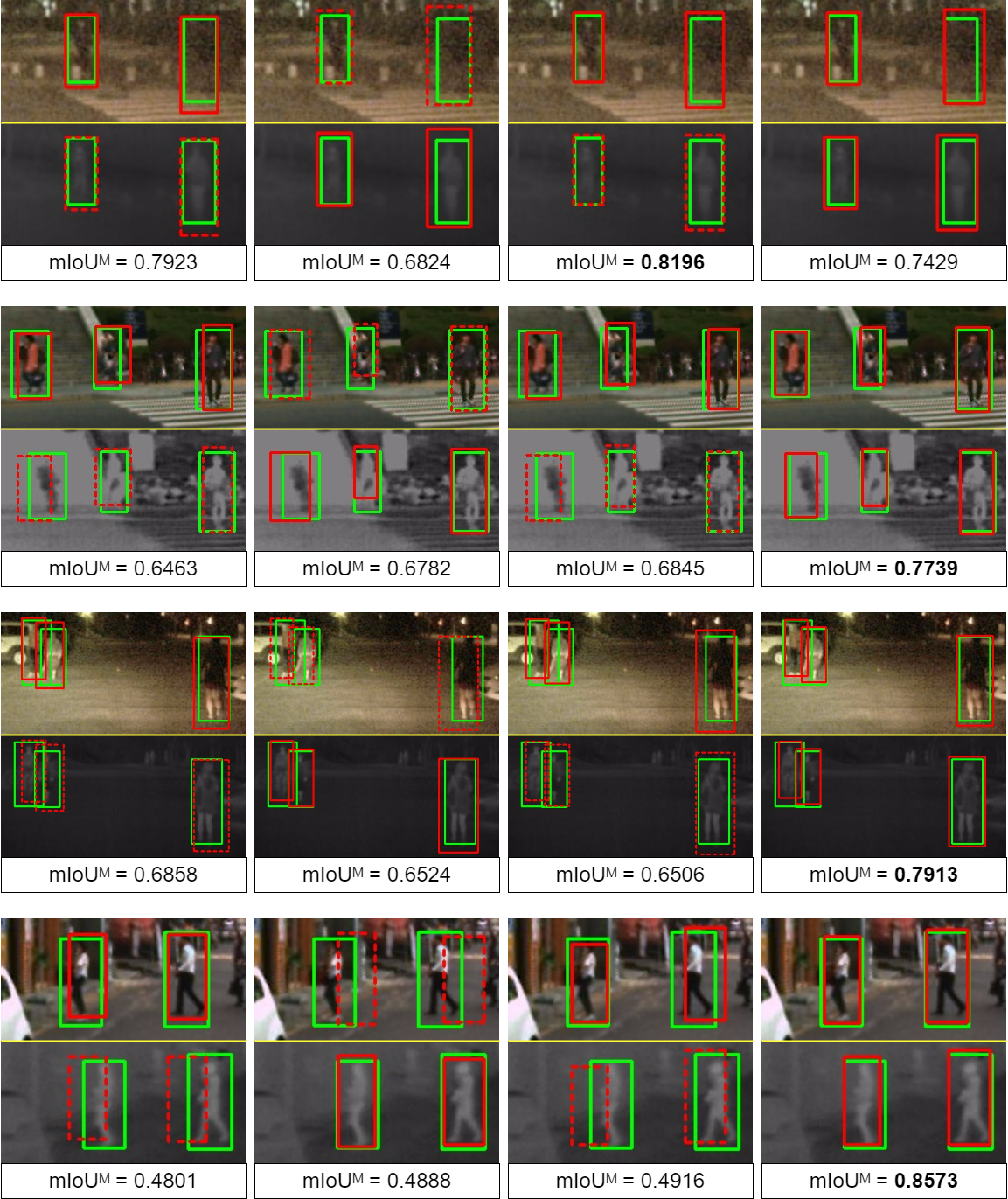
Qualitative comparison examples of detection results on KAIST dataset [2] of MSDS-RCNN [3],
AR-CNN [4], MBNet [5], and ours. Green bounding boxes represent ground truth
by Lu Zhang et al. [4], and red bounding boxes represent detection results. Dashed line bounding
boxes denote substituted bounding boxes for methods that do not have paired bounding boxes.
References
[1] Faster R-CNN: Shaoqing Ren, Kaiming He, Ross Girshick, and Jian Sun, “Faster R-CNN: Towards Real-Time Object Detection with Region Proposal Networks,” in Proceedings of the IEEE Transactions on Pattern Analysis and Machine Intelligence, vol. 39, no. 6, pp. 1137-1149, 2017.
[2] KAIST dataset: Soonmin Hwang, Jaesik Park, Namil Kim, Yukyung Choi, and In So Kweon, “Multispectral Pedestrian Detection: Benchmark Dataset and Baseline,” in Proceedings of the IEEE Conference on Computer Vision and Pattern Recognition (CVPR), 2015.
[3] MSDS-RCNN: Chengyang Li, Dan Song, Ruofeng Tong, and Min Tang, “Multispectral Pedestrian Detection via Simultaneous Detection and Segmentation,” in Proceedings of the British Machine Vision Conference (BMVC), 2018.
[4] AR-CNN: Lu Zhang, Xiangyu Zhu, Xiangyu Chen, Xu Yang, Zhen Lei, and Zhiyong Liu, “Weakly Aligned Cross-Modal Learning for Multispectral Pedestrian Detection,” in Proceedings of the IEEE International Conference on Computer Vision (ICCV), 2019.
[5] MBNet: Kailai Zhou, Linsen Chen, and Xun Cao, “Improving Multispectral Pedestrian Detection by Addressing Modality Imbalance Problems,” in Proceedings of the European Conference on Computer Vision (ECCV), pages 787-803, 2020.
Publication
- Multi-Modal Pedestrian Detection with Large Misalignment Based on Modal-Wise Regression and Multi-Modal IoU [arXiv]
- Napat Wanchaitanawong, Masayuki Tanaka, Takashi Shibata, and Masatoshi Okutomi
- Proceedings of the 17th International Conference on Machine Vision Applications (MVA2021), pp.O1-1-4-1-6, July 2021.
- Multi-Modal Pedestrian Detection with Large Misalignment Based on Modal-Wise Regression and Multi-Modal IoU [SPIE]
- Napat Wanchaitanawong, Masayuki Tanaka, Takashi Shibata, and Masatoshi Okutomi
- Journal of Electronic Imaging, Vol.32, Issue 1, pp.013025-1-19, February 2023.