Multi-Modal Pedestrian Detection via Dual-Regressor and
Object-Based Training for One-Stage Object Detection Network
Contributions
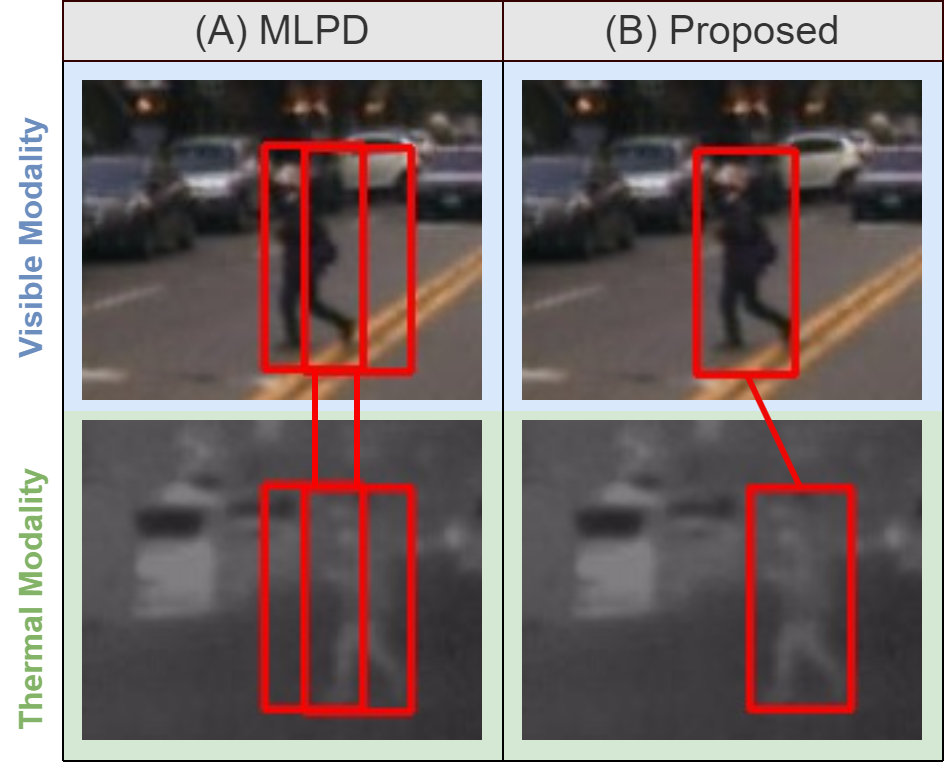
We proposed multi-modal dual-regressor for one-stage detector.
We proposed object-based training for paired annotations of multi-modal data.
We proposed shifting data augmentation to train multi-modal network against misalignment.
Proposed Network Overview
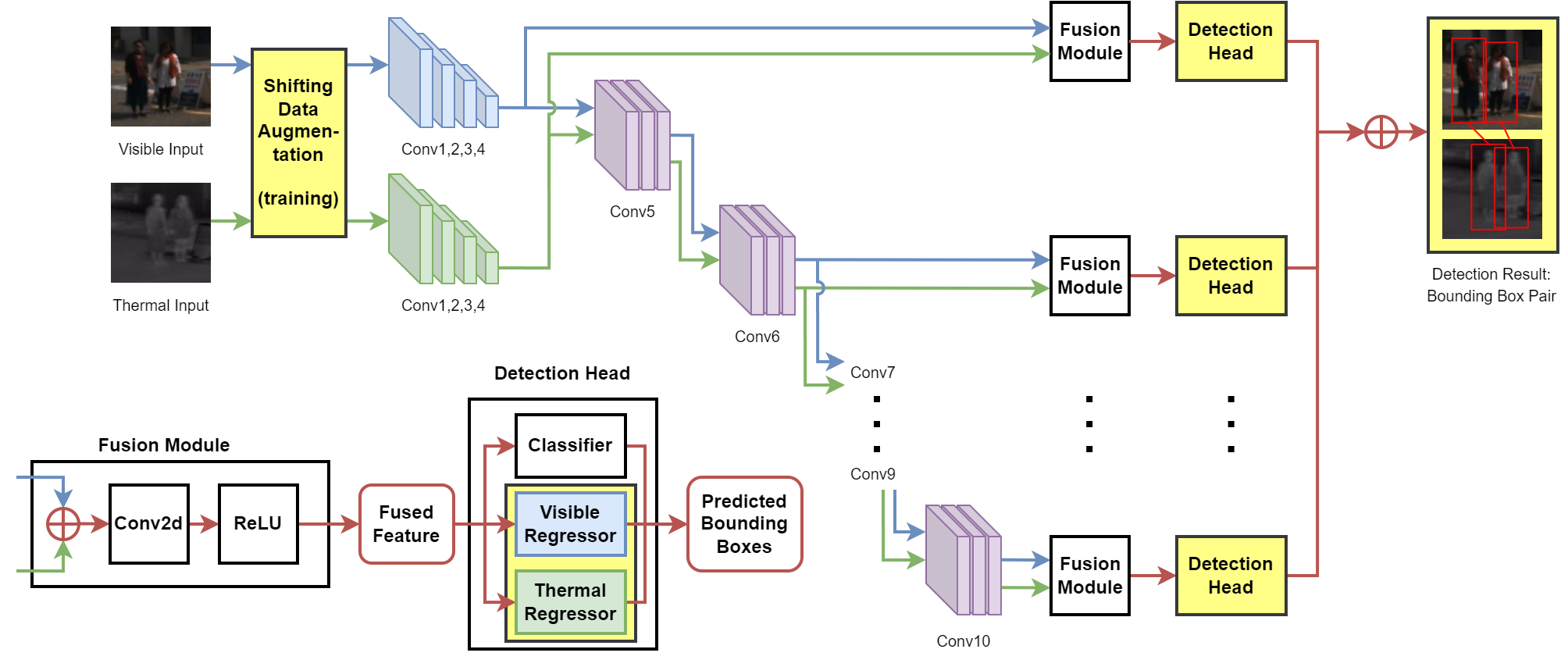
The overall architecture of our network.
The framework is based on SSD [1] customized by MLPD [2].
Yellow blocks represent
notable changes introduced in our method: shifting data augmentation in the training phase, detection heads with visible regressors and
thermal regressors, and detection outputs consisting of pairs of bounding boxes.
Blue, green, and red blocks/paths represent properties of
visible modality, thermal modality, and fused modalities, respectively.
⊕ denotes channel-wise concatenation.
Visualization Examples
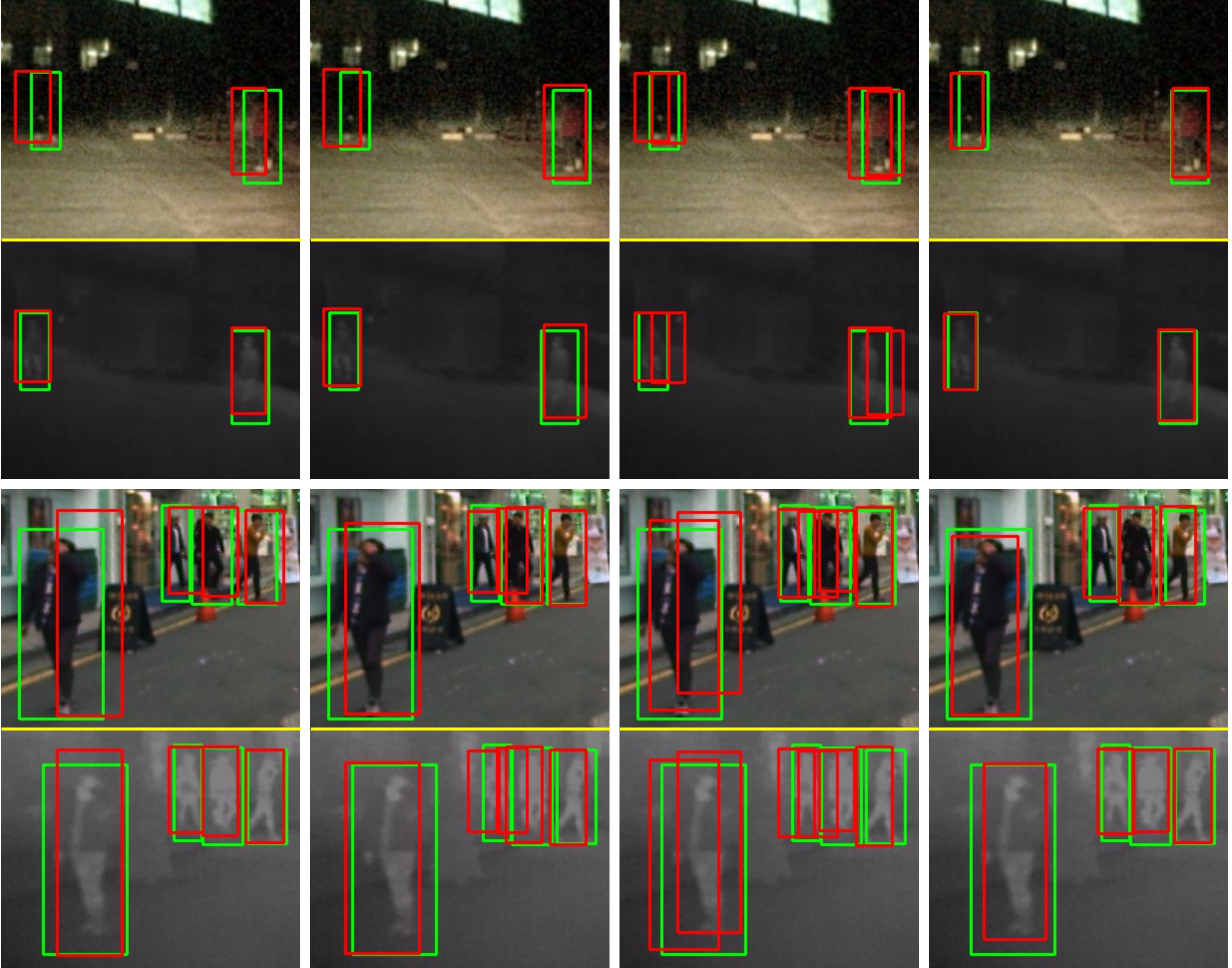
Qualitative comparison examples of detection results on KAIST dataset [3] of AR-CNN [4], MBNet [5], MLPD [2], and ours.
Green boxes represent ground truth bounding boxes.
Red boxes represent predicted bounding boxes.
Image pairs are cropped in the same position to make the contrast between methods more apparent.
References
[1] SSD: Wei Liu, Dragomir Anguelov, Dumitru Erhan, Christian Szegedy, Scott Reed, Cheng-Yang Fu, and Alexander C. Berg, "SSD: Single Shot Multibox Detector," in proceedings of the European Conference on Computer Vision (ECCV), Springer, 2016.
[2] MLPD: Jiwon Kim, Hyeongjun Kim, Taejoo Kim, Namil Kim, and Yukyung Choi, “MLPD: Multi-Label Pedestrian Detector in Multispectral Domain,” in IEEE Robotics and Automation Letters, vol. 6, no. 4, pp. 7846-7853, Oct. 2021.
[3] KAIST dataset: Soonmin Hwang, Jaesik Park, Namil Kim, Yukyung Choi, and In So Kweon, “Multispectral Pedestrian Detection: Benchmark Dataset and Baseline,” in proceedings of IEEE Conference on Computer Vision and Pattern Recognition (CVPR), 2015.
[4] AR-CNN: Lu Zhang, Xiangyu Zhu, Xiangyu Chen, Xu Yang, Zhen Lei, and Zhiyong Liu, "Weakly Aligned Cross-Modal Learning for Multispectral Pedestrian Detection,” in proceedings of the IEEE International Conference on Computer Vision (ICCV), 2019.
[5] MBNet: Kailai Zhou, Linsen Chen, and Xun Cao, "Improving Multispectral Pedestrian Detection by Addressing Modality Imbalance Problems,” in proceedings of the European Conference on Computer Vision (ECCV), pages 787-803, 2020.
Publication
- Multi-Modal Pedestrian Detection via Dual-Regressor and Object-Based Training for One-Stage Object Detection Network
[Paper]
[GitHub]
- Napat Wanchaitanawong, Masayuki Tanaka, Takashi Shibata, and Masatoshi Okutomi
- Electronic Imaging (EI2024), January 2024.