Instance-Wise MRI Reconstruction Based on Self-Supervised Implicit Neural Representation
Songxiao Yang, Yizhou Li, and Masatoshi Okutomi
Department of Systems and Control Engineering, School of Engineering, Institute of Science Tokyo
46th Annual International Conference of the IEEE Engineering in Medicine and Biology Society (EMBC, Oral)
Abstract
Accelerated MRI involves a trade-off between sampling sufficiency and acquisition time. Supervised deep learning methods have shown great success in MRI reconstruction from under-sampled measurements, but they typically require a large set of fully-sampled MR images for training, which can be difficult to obtain. In this paper, we present a novel fully self-supervised method based on implicit neural representation, which requires only a single under-sampled MRI instance for training. To effectively guide the self-supervised learning process, we introduced multiple novel supervisory signals in both the image and frequency domains. Experimental results indicate that the proposed method outperforms existing self-supervised methods and even a supervised method, demonstrating its strong reliability and flexibility.
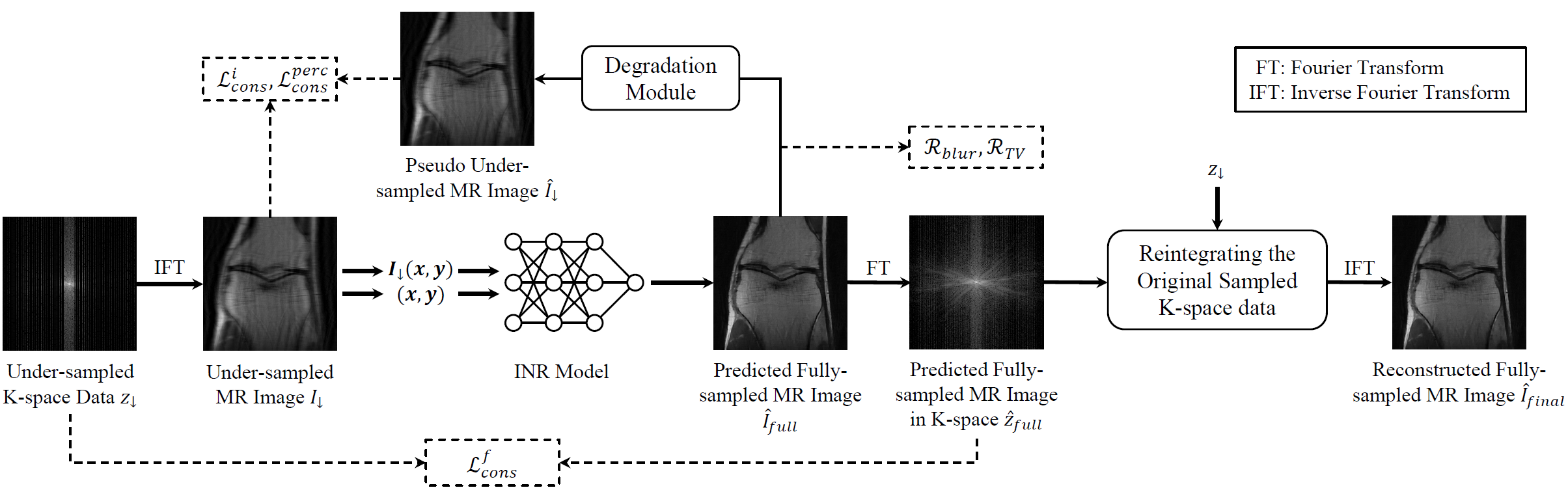
Visual Comparisons
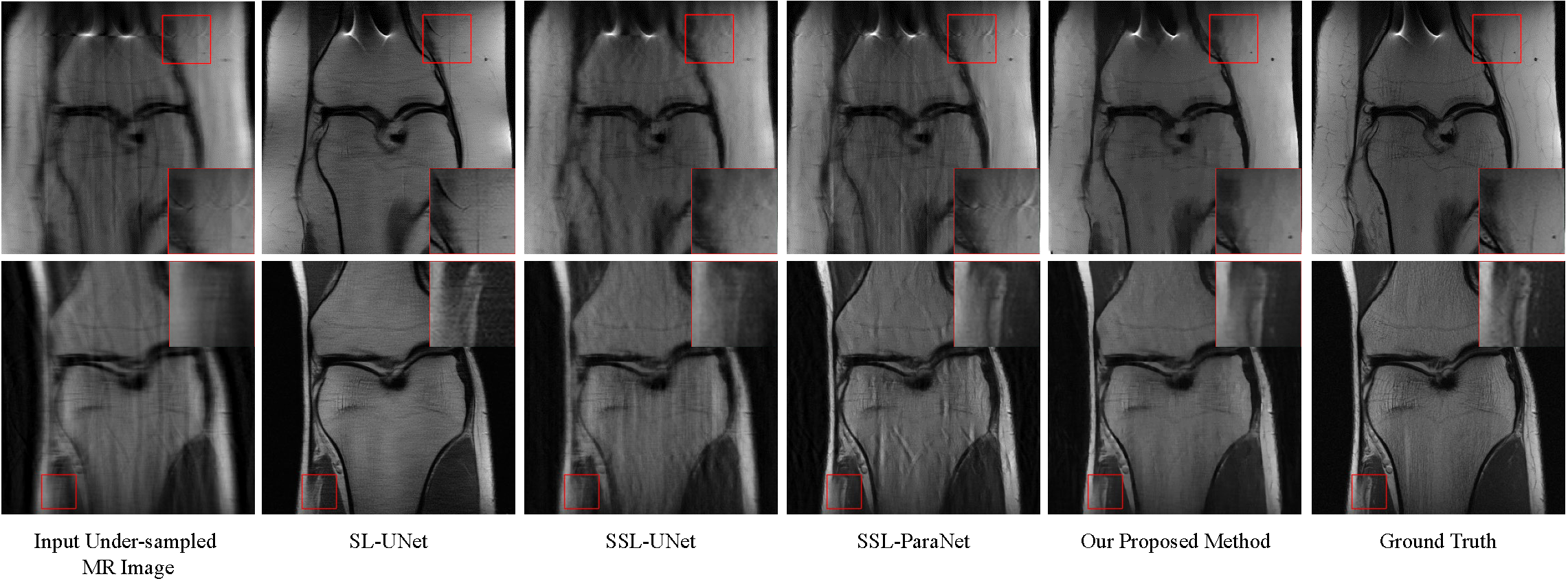
Publication
Instance-Wise MRI Reconstruction Based on Self-Supervised Implicit Neural Representation
Authors: Songxiao Yang, Yizhou Li, and Masatoshi Okutomi
46th Annual International Conference of the IEEE Engineering in Medicine and Biology Society (EMBC2024, Oral)
@inproceedings{yang2024instance,
title={Instance-Wise MRI Reconstruction Based on Self-Supervised Implicit Neural Representation},
author={Yang, Song-Xiao and Li, Yi-Zhou and Okutomi, Masatoshi},
booktitle={2024 46th Annual International Conference of the IEEE Engineering in Medicine and Biology Society (EMBC)},
pages={1--4},
year={2024},
organization={IEEE}
}